What is weighted covariance?
Table of Contents
What is weighted covariance?
A weighted covariance allows you to apply a weight, or relative significance to each value comparison. Covariance comparisons with a higher value for their weight are considered as more significant when compared to the other value comparisons.
What is the meaning of a covariance matrix?
In probability theory and statistics, a covariance matrix (also known as auto-covariance matrix, dispersion matrix, variance matrix, or variance–covariance matrix) is a square matrix giving the covariance between each pair of elements of a given random vector.
What does a variance-covariance matrix tell you?
The variance-covariance matrix expresses patterns of variability as well as covariation across the columns of the data matrix. In most contexts the (vertical) columns of the data matrix consist of variables under consideration in a study and the (horizontal) rows represent individual records.

What is the difference between correlation matrix and covariance matrix?
Covariance and correlation are two terms that are opposed and are both used in statistics and regression analysis. Covariance shows you how the two variables differ, whereas correlation shows you how the two variables are related.
What is weighted correlation?
A weighted correlation allows you to apply a weight, or relative significance to each value comparison. Correlation comparisons with a higher value for their weight are considered as more significant when compared to the other value comparisons.
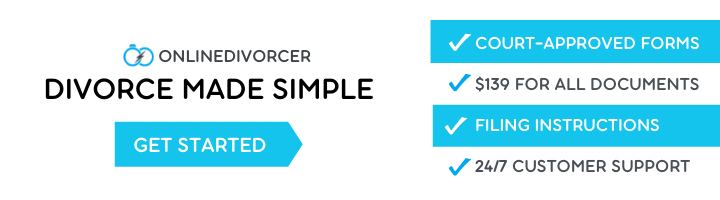
What is the difference between population covariance and sample covariance?
The only difference in formula for Population Covariance and Sample Covariance lies in the fact that Population Covariance is calculated over the entire dataset(N) whereas Sample Covariance is calculated over a sample (N-1), so that the denominator of the Population Covariance is 1 larger than that of the Sample …
How do you write a covariance matrix?
Here’s how.
- Transform the raw scores from matrix X into deviation scores for matrix x. x = X – 11’X ( 1 / n )
- Compute x’x, the k x k deviation sums of squares and cross products matrix for x.
- Then, divide each term in the deviation sums of squares and cross product matrix by n to create the variance-covariance matrix.
What is difference between variance and covariance?
Variance and covariance are mathematical terms frequently used in statistics and probability theory. Variance refers to the spread of a data set around its mean value, while a covariance refers to the measure of the directional relationship between two random variables.
Why is it called variance-covariance matrix?
A variance-covariance matrix is a square matrix that contains the variances and covariances associated with several variables. The diagonal elements of the matrix contain the variances of the variables and the off-diagonal elements contain the covariances between all possible pairs of variables.
What is Wgcna used for?
Weighted gene co-expression network analysis (WGCNA) is a bioinformatics application for exploring the relationships between different gene sets (modules), or between gene sets and clinical features (Langfelder and Horvath, 2008).
What is weighted network analysis?
Weighted correlation network analysis, also known as weighted gene co-expression network analysis (WGCNA), is a widely used data mining method especially for studying biological networks based on pairwise correlations between variables.
What is the difference between variance and covariance?
What is population covariance?
The population covariance between and is obtained by summing over all pairs of variables. We then multiply respective coefficients from the two linear combinations as times times the covariances between j and k. Population Covariance between two linear combinations.
What is covariance used for?
Covariance is a statistical tool that is used to determine the relationship between the movements of two random variables. When two stocks tend to move together, they are seen as having a positive covariance; when they move inversely, the covariance is negative.
Can covariance matrix have negative values?
Unlike Variance, which is non-negative, Covariance can be negative or positive (or zero, of course). A positive value of Covariance means that two random variables tend to vary in the same direction, a negative value means that they vary in opposite directions, and a 0 means that they don’t vary together.
Why do we need covariance matrix?
The covariance matrix provides a useful tool for separating the structured relationships in a matrix of random variables. This can be used to decorrelate variables or applied as a transform to other variables. It is a key element used in the Principal Component Analysis data reduction method, or PCA for short.
Is a covariance matrix always square?
Also, Variance-Covariance matrices are always square matrices of size n, where n is the number of variables in your experiment.