What is quasi-likelihood approach?
Table of Contents
What is quasi-likelihood approach?
In statistics, quasi-likelihood methods are used to estimate parameters in a statistical model when exact likelihood methods, for example maximum likelihood estimation, are computationally infeasible.
What is the maximum likelihood strategy?
In statistics, maximum likelihood estimation (MLE) is a method of estimating the parameters of an assumed probability distribution, given some observed data. This is achieved by maximizing a likelihood function so that, under the assumed statistical model, the observed data is most probable.
What is penalized quasi-likelihood?
The Penalized Quasi Likelihood (PQL) method has been proposed to fit generalized linear mixed-effects models. The way it works is by doing a kind of a Laplace approximation in a quasi-likelihood formulation of the model. This approximation results in a transformation of the original outcome variable.

What quasi binomial?
The quasi-binomial (QBD) model has two parameters p and ϕ. The parameter p will be called the binomial parameter and the other parameter ϕ will be called the dispersion parameter. When ϕ=0, the quasi-binomial distribution (QBD) reduces to the binomial distribution.
What is quasi regression?
Abstract. Quasi-regression is introduced for approximation of functions on the unit cube in s dimensions. It is computationally efficient, compared to kriging, for problems requiring a large number of function evaluations.
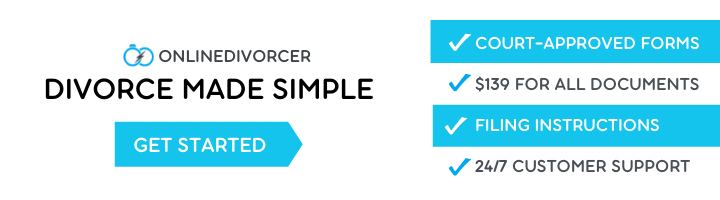
What is the main disadvantage of maximum likelihood methods?
Explanation: The main disadvantage of maximum likelihood methods is that they are computationally intense. However, with faster computers, the maximum likelihood method is seeing wider use and is being used for more complex models of evolution.
What is the difference between Bayesian estimation and MLE?
This is the difference between MLE/MAP and Bayesian inference. MLE and MAP returns a single fixed value, but Bayesian inference returns probability density (or mass) function.
Why maximum likelihood estimation is used?
Maximum likelihood estimation involves defining a likelihood function for calculating the conditional probability of observing the data sample given a probability distribution and distribution parameters. This approach can be used to search a space of possible distributions and parameters.
What is a quasi Poisson model?
The Quasi-Poisson Regression is a generalization of the Poisson regression and is used when modeling an overdispersed count variable. The Poisson model assumes that the variance is equal to the mean, which is not always a fair assumption.