What is the central limit theorem in statistics?
Table of Contents
What is the central limit theorem in statistics?
The central limit theorem states that if you have a population with mean μ and standard deviation σ and take sufficiently large random samples from the population with replacement , then the distribution of the sample means will be approximately normally distributed.
What is a Cauchy distribution in statistics?
The Cauchy distribution, also called the Lorentzian distribution or Lorentz distribution, is a continuous distribution describing resonance behavior. It also describes the distribution of horizontal distances at which a line segment tilted at a random angle cuts the x-axis.
Why is central limit theorem important in statistics?
The Central Limit Theorem is important for statistics because it allows us to safely assume that the sampling distribution of the mean will be normal in most cases. This means that we can take advantage of statistical techniques that assume a normal distribution, as we will see in the next section.
What is central limit theorem for sampling distributions?

The Central Limit Theorem states that the sampling distribution of the sample means approaches a normal distribution as the sample size gets larger — no matter what the shape of the population distribution. This fact holds especially true for sample sizes over 30.
What is the characteristic function of Cauchy distribution?
But of course the characteristic function of the Cauchy distribution exists and is easy to obtain from the characteristic function of the standard distribution. has characteristic function given by χ ( t ) = exp ( a i t − b | t | ) for t ∈ R .
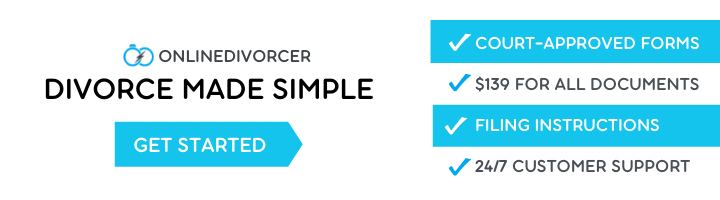
How is the central limit theorem used in real life?
Biologists use the central limit theorem whenever they use data from a sample of organisms to draw conclusions about the overall population of organisms. For example, a biologist may measure the height of 30 randomly selected plants and then use the sample mean height to estimate the population mean height.
How do you know when to use the central limit theorem?
If you are being asked to find the probability of the mean, use the clt for the mean. If you are being asked to find the probability of a sum or total, use the clt for sums. This also applies to percentiles for means and sums. If you are being asked to find the probability of an individual value, do not use the clt.
Does the central limit theorem apply to all distributions?
The central limit theorem applies to almost all types of probability distributions, but there are exceptions. For example, the population must have a finite variance.
How do you prove a Cauchy distribution?
Proof: Recall that F ( x ) = G ( x − a b ) where G is the standard Cauchy CDF. has quantile function given by F − 1 ( p ) = a + b tan [ π ( p − 1 2 ) ] , p ∈ ( 0 , 1 ) In particular, The first quartile is F − 1 ( 1 4 ) = a − b . The median is F − 1 ( 1 2 ) = a . The third quartile is F − 1 ( 3 4 ) = a + b .
How is CLT related to sampling distribution of the sampling means?
What are the implications of the central limit theorem?
Central limit theorem helps us to make inferences about the sample and population parameters and construct better machine learning models using them. Moreover, the theorem can tell us whether a sample possibly belongs to a population by looking at the sampling distribution.
How does the central limit theorem allow us to make inferences about a population?
The CLT reveals exactly what the shape of the mean distribution will be when repeated samples are drawn from a given population. More specifically, as sample sizes become larger, the mean distribution that is measured from repeated sampling will reach the normal limits.
When can we use the central limit theorem?
It is important for you to understand when to use the central limit theorem. If you are being asked to find the probability of the mean, use the clt for the mean. If you are being asked to find the probability of a sum or total, use the clt for sums. This also applies to percentiles for means and sums.
Which conditions must be met for the central limit theorem to apply?
Assumptions Behind the Central Limit Theorem
- The data must follow the randomization condition. It must be sampled randomly.
- Samples should be independent of each other.
- Sample size should be not more than 10% of the population when sampling is done without replacement.
- The sample size should be sufficiently large.