What is a random effect in a model?
Table of Contents
What is a random effect in a model?
In econometrics, random effects models are used in panel analysis of hierarchical or panel data when one assumes no fixed effects (it allows for individual effects). A random effects model is a special case of a mixed model.
What is a random effects estimator?
The Random Effects regression model is used to estimate the effect of individual-specific characteristics such as grit or acumen that are inherently unmeasurable. Such individual-specific effects are often encountered in panel data studies.
What is random effect in mixed model?
Random effects are simply the extension of the partial pooling technique as a general-purpose statistical model. This enables principled application of the idea to a wide variety of situations, including multiple predictors, mixed continuous and categorical variables, and complex correlation structures.

Why do you use random effects model?
The random-effects model allows making inferences on the population data based on the assumption of normal distribution. The random-effects model assumes that the individual-specific effects are uncorrelated with the independent variables.
What is the difference between fixed effect and random effect?
The fixed effects are the coefficients (intercept, slope) as we usually think about the. The random effects are the variances of the intercepts or slopes across groups. In the HLM program, variances for the intercepts and slopes are estimated by default (U0j and U1j, respectively).
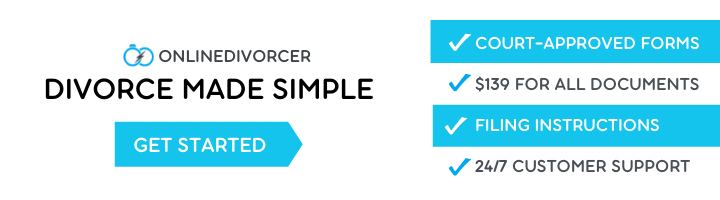
Why do we use random effect model?
What is random effect model in meta-analysis?
Random effects meta-analysis A random-effects meta-analysis model assumes the observed estimates of treatment effect can vary across studies because of real differences in the treatment effect in each study as well as sampling variability (chance).
What is the difference between fixed effect and random effect models?
The fixed-effects model assumes that the individual-specific effect is correlated to the independent variable. The random-effects model allows making inferences on the population data based on the assumption of normal distribution.
What is the difference between fixed-effects and random effects?
A fixed-effects model supports prediction about only the levels/categories of features used for training. A random-effects model, by contrast, allows predicting something about the population from which the sample is drawn.
What is the difference between random and fixed-effects meta-analysis models?
A random-effects model assumes each study estimates a different underlying true effect, and these effects have a distribution (usually a normal distribution). Fixed-effects model should be used only if it reasonable to assume that all studies shares the same, one common effect.
Why do we use the random effect model?
Should I use fixed or random effects meta-analysis?
Fixed-effects model should be used only if it reasonable to assume that all studies shares the same, one common effect. If it is not reasonable to assume that there is one common effect size, then the random-effects model should be used.
How do I create a random-effects model using theta?
Note that once theta is known, this model can be created using the PANEL_DEMEAN function described in Demeaning for Panel Data by setting the weight parameter to theta. The random-effects model includes support for time-invariant independent variables xij (i.e. where xitj = xisj for all times t, s).
What is the random effects model?
Along with the Fixed Effect regression model, the Random Effects model is a commonly used technique to study the effect of individual-specific features on the response variable of the panel data set. This article is PART 3 of the following three part series on Panel Data Analysis:
What is the difference between fixed and random effects?
The random effects model is a special case of the fixed effects model. The random effects assumption is that the individual specific effects are uncorrelated with the independent variables.
Does the random-effects model predict valuing health states?
The use of the random-effects model demonstrated that there were significant differences between respondents regarding their overall level of valuing health states ( p < .001). This same model resulted in coefficients similar to the coefficients of a standard regression model. However, the standard errors are smaller for the random-effects model.