Can SPSS do confirmatory factor analysis?
Table of Contents
Can SPSS do confirmatory factor analysis?
SPSS does not include confirmatory factor analysis but those who are interested could take a look at AMOS.
What does confirmatory factor analysis tell you?
Confirmatory factor analysis (CFA) is a statistical technique used to verify the factor structure of a set of observed variables. CFA allows the researcher to test the hypothesis that a relationship between observed variables and their underlying latent constructs exists.
How do you present confirmatory factor analysis results?
Each row should contain the results of a different model, with lower-factor models above higher-factor models. The first row should contain each model’s name; rows to the left contain chi-square value, degrees of freedom, goodness-of-fit index and any other important data. Label each column in your heading row.

Can we run CFA in SPSS?
You need to purchase the Analysis of Moment Structue {AMOS} to rund CFA. You can not use SPSS. You can use AMOS, LISREL or MPlus.
How do you interpret a factor analysis?
- Step 1: Determine the number of factors. If you do not know the number of factors to use, first perform the analysis using the principal components method of extraction, without specifying the number of factors.
- Step 2: Interpret the factors.
- Step 3: Check your data for problems.
How do you use confirmatory factor analysis?
Steps in a Confirmatory Factor Analysis. The first step is to calculate the factor loadings of the indicators (observed variables) that make up the latent construct. The standardized factor loading squared is the estimate of the amount of the variance of the indicator that is accounted for by the latent construct.
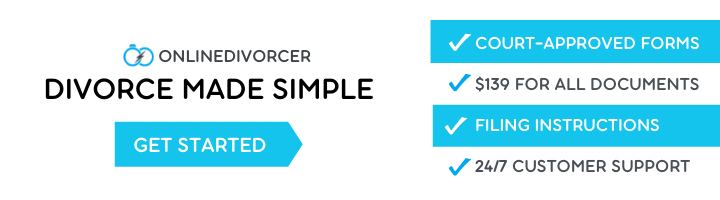
What is CFA test in SPSS?
In confirmatory factor analysis (CFA), you specify a model, indicating which variables load on which factors and which factors are correlated. You would get a measure of fit of your data to this model.
What is a good eigenvalue?
Eigenvalues represent the total amount of variance that can be explained by a given principal component. They can be positive or negative in theory, but in practice they explain variance which is always positive. If eigenvalues are greater than zero, then it’s a good sign.
What is an acceptable CFI?
CFI values range from 0 to 1, with larger values indicating better fit. Previously, a CFI value of . 90 or larger was considered to indicate acceptable model fit.
How do you interpret eigenvalues in factor analysis?
What is a good CFI?
06 and a CFI and TLI larger than . 95 indicate relatively good model–data fit in general. Hu and Bentler’s study has become highly influential, and their recommended cutoffs have been adopted in many SEM practices.
What is the meaning of eigenvalue 1 in factor analysis?
The eigenvalue is a measure of how much of the common variance of the observed variables a factor explains. Any factor with an eigenvalue ≥1 explains more variance than a single observed variable.
What does eigenvalue less than 1 mean?
An eigenvalue less than 1 means that the PC explains less than a single original variable explained, i.e. it has no value, the original variable was better than the new variable PC2. This would fit with factor rotation producing a second factor that is related to a single variable.
What does a zero eigenvalue mean?
If an eigenvalue of A is zero, it means that the kernel (nullspace) of the matrix is nonzero. This means that the matrix has determinant equal to zero. Such a matrix will not be invertible.