How do you calculate pure error?
Table of Contents
How do you calculate pure error?
Pure error reflects the variability of the observations within each treatment. The sum of squares for the pure error is the sum of the squared deviations of the responses from the mean response in each set of replicates.
What are the degrees of freedom for the error sum of squares?
The degrees of freedom for the sum of squares explained is equal to the number of predictor variables. This will always be 1 in simple regression. The error degrees of freedom is equal to the total number of observations minus 2.
How do you calculate degrees of freedom for residuals?
The df(Residual) is the sample size minus the number of parameters being estimated, so it becomes df(Residual) = n – (k+1) or df(Residual) = n – k – 1. It’s often easier just to use subtraction once you know the total and the regression degrees of freedom.

What is lack of fit error?
∙ Lack of fit error: Error that occurs when the analysis omits one or more important terms or factors from the process model. ∙ Pure error: I occurs for repeated values of dependent variable, Y for a fixed value of independent variable, X.
What is the degree of freedom for error?
The degrees of freedom add up, so we can get the error degrees of freedom by subtracting the degrees of freedom associated with the factor from the total degrees of freedom. That is, the error degrees of freedom is 14−2 = 12.
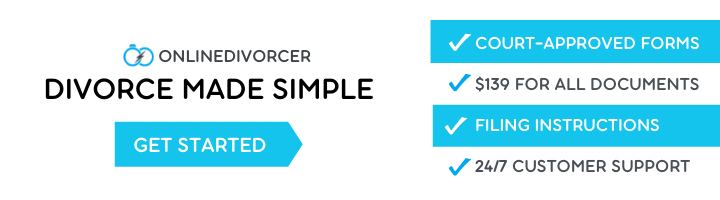
How do you calculate degree of freedom?
To calculate degrees of freedom, subtract the number of relations from the number of observations. For determining the degrees of freedom for a sample mean or average, you need to subtract one (1) from the number of observations, n.
How do you calculate lack of fit?
Analysis of Variance You might notice that the lack of fit F-statistic is calculated by dividing the lack of fit mean square (MSLF = 3398) by the pure error mean square (MSPE = 230) to get 14.80.
Why lack of fit is significant?
A lack-of-fit error significantly larger than the pure error indicates that something remains in the residuals that can be removed by a more appropriate model. If you see significant lack-of-fit (Prob>F value 0.10 or smaller) then don’t use the model as a predictor of the response.
What is the degree of freedom for F-test?
The distribution used for the hypothesis test is a new one. It is called the F distribution, named after Sir Ronald Fisher, an English statistician. The F statistic is a ratio (a fraction). There are two sets of degrees of freedom; one for the numerator and one for the denominator.
What is the degree of freedom for F test?
How do you find degrees of freedom?
What is df error in statistics?
What is degree of freedom example?
For example, let’s say you had 200 observations and four cell means. Degrees of freedom in this case would be: Df2 = 200 – 4 = 196.
What is the degree of freedom of any t statistic calculated?
Degrees of Freedom for t Tests We know that when you have a sample and estimate the mean, you have n – 1 degrees of freedom, where n is the sample size. Consequently, for a 1-sample t test, use n – 1 to calculate degrees of freedom.
How do you calculate degrees of freedom for pure error?
In other words, the degrees of freedom for pure error will equal: m is the number of corner points in the model r is the number of replicates c is the number of center points The sum of squares for pure error is the sum of the squared deviations of the responses from the mean response in each set of replicates.
How do you find the lack of fit degrees of freedom?
The lack-of-fit degrees of freedom is found by subtracting the degrees of freedom for pure error and curvature (if appropriate) from the residual-error degrees of freedom. The sum of squares for lack of fit is found by subtracting the sums of squares for pure error and curvature (if appropriate) from the residual-error sum of squares.
What are the degrees of freedom associated with the sum-of-squares?
The degrees of freedom associated with a sum-of-squares is the degrees-of-freedom of the corresponding component vectors. The three-population example above is an example of one-way Analysis of Variance. The model, or treatment, sum-of-squares is the squared length of the second vector,
What is the sum of squares due to pure error?
The sum of squares due to “pure” error is the sum of squares of the differences between each observed y -value and the average of all y -values corresponding to the same x -value.