Is the maximum likelihood estimator consistent?
Table of Contents
Is the maximum likelihood estimator consistent?
It is shown that, under usual regularity conditions, the maximum likelihood estimator of a structural parameter is strongly consistent, when the (infinitely many) incidental parameters are independently distributed chance variables with a common unknown distribution function.
Is maximum likelihood always consistent?
Ultimately, we will show that the maximum likelihood estimator is, in many cases, asymptotically normal. However, this is not always the case; in fact, it is not even necessarily true that the MLE is consistent, as shown in Problem 27.1.
Why is the maximum likelihood estimator a preferred estimator?
To answer your question of why the MLE is so popular, consider that although it can be biased, it is consistent under standard conditions. In addition, it is asymptotically efficient, so at least for large samples, the MLE is likely to do as well or better as any other estimator you may cook up.

Does maximum likelihood estimator always exist?
If the interval included its boundary, then clearly the MLE would be θ = max[Xi]. But since this interval does not include its boundary, the MLE cannot be the maximum, and therefore an MLE does not exist.
How do you prove MLE is unbiased?
It is easy to check that the MLE is an unbiased estimator (E[̂θMLE(y)] = θ). To determine the CRLB, we need to calculate the Fisher information of the model. Yk) = σ2 n . (6) So CRLB equality is achieved, thus the MLE is efficient.
What is the purpose of maximum likelihood estimation?
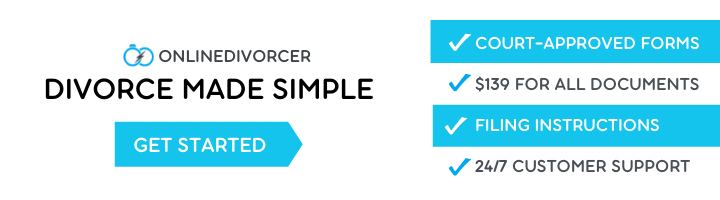
Maximum Likelihood Estimation is a probabilistic framework for solving the problem of density estimation. It involves maximizing a likelihood function in order to find the probability distribution and parameters that best explain the observed data.
Which of the following is true about maximum likelihood estimate?
Q3. Which of the following is/ are true about “Maximum Likelihood estimate (MLE)”? Solution: CThe MLE may not be a turning point i.e. may not be a point at which the first derivative of the likelihood (and log-likelihood) function vanishes.
How do you prove MLE is unique?
Proposition 3 (Sufficient condition for uniqueness of MLE) If the parameter space Θ is convex and if the likelihood function θ ↦→ l (y; θ) is strictly concave in θ, then the MLE is unique when it exists.
What are the assumptions for using maximum likelihood estimators?
In order to use MLE, we have to make two important assumptions, which are typically referred to together as the i.i.d. assumption. These assumptions state that: Data must be independently distributed. Data must be identically distributed.
Is maximum likelihood estimator biased?
It is well known that maximum likelihood estimators are often biased, and it is of use to estimate the expected bias so that we can reduce the mean square errors of our parameter estimates.
What is MLE and its properties?
Maximum Likelihood Estimation (MLE) is a widely used statistical estimation method. In this lecture, we will study its properties: efficiency, consistency and asymptotic normality. MLE is a method for estimating parameters of a statistical model.
Which of the following is wrong statement about maximum likelihood estimation?
7. Which of the following is wrong statement about the maximum likelihood method’s steps? Explanation: The rates of all possible substitutions are chosen so that the base composition remains the same.
How do you derive the maximum likelihood estimator?
STEP 1 Calculate the likelihood function L(λ). log(xi!) STEP 3 Differentiate logL(λ) with respect to λ, and equate the derivative to zero to find the m.l.e.. Thus the maximum likelihood estimate of λ is ̂λ = ¯x STEP 4 Check that the second derivative of log L(λ) with respect to λ is negative at λ = ̂λ.
Which of the following models can be estimated by maximum likelihood estimator?
Which of the following models can be estimated by maximum likelihood estimator? (d) Naive Bayes. In Naïve Bayes, the parameters q(y) and q(x|y) can be estimated from data using maximum likelihood estimation.
What is the difference between Bayesian inference and maximum likelihood estimation MLE )?
This is the difference between MLE/MAP and Bayesian inference. MLE and MAP returns a single fixed value, but Bayesian inference returns probability density (or mass) function.
Is MLE Bayesian or frequentist?
The accepted answer either links maximum likelihood point estimation stronger to the frequentist risk or provides an alternative formal definition of frequentist inference that shows that MLE is a frequentist inference technique.