Why is it more appropriate to use a log model?
Table of Contents
Why is it more appropriate to use a log model?
Using the logarithm of one or more variables improves the fit of the model by transforming the distribution of the features to a more normally-shaped bell curve.
Why log-linear model is useful for economists?
If you use natural log values for your dependent variable (Y) and keep your independent variables (X) in their original scale, the econometric specification is called a log-linear model. These models are typically used when you think the variables may have an exponential growth relationship.
What is the main advantage of log linear models over linear regression?
The two great advantages of log-linear models are that they are flexible and they are interpretable. Log-linear models have all the flexibility associated with ANOVA and regression. We have mentioned before that log-linear models are also another form of GLM.

What is a log log regression model?
A regression model where the outcome and at least one predictor are log transformed is called a log-log linear model.
How do you choose between linear and log-linear models?
Answer: The linear or log-linear model can be chosen depending on how linear the observed rates or the logarithm of the observed rates are over time. In order to check the goodness of fit of the chosen model, a user can test for normality of the residuals obtained under the linear or the log-linear fit.
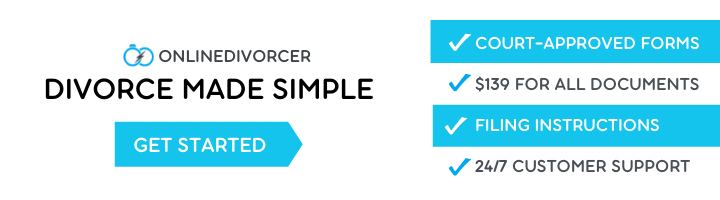
Why is logit better than LPM?
So even with this data generating process that is not directly congenial to logit, the bounded range of the probability means that the logit model gives much more consistent results than the LPM.
How do you choose between linear and log linear models?
When should you use the log of a variable?
Log can be used in 2 instances, (i) when you need to interpret your results in percent changes or elasticities and (ii) to bring all variables to the same level (thereby getting rid of outliers in the process).
When should you log transform data?
When our original continuous data do not follow the bell curve, we can log transform this data to make it as “normal” as possible so that the statistical analysis results from this data become more valid . In other words, the log transformation reduces or removes the skewness of our original data.
When we use log linear regression?
Thus we see that in practice we should use a log-linear model when dependent and independent variables have lognormal distributions. On the other hand, when those variables are normal or close to normal, we should rather stay with a simple linear model.
When should I use logit?
Use logit models whenever your dependent variable is binary (also called dummy) which takes values 0 or 1. Logit regression is a nonlinear regression model that forces the output (predicted values) to be either 0 or 1.
Which is better probit or logit?
Probit is better in the case of “random effects models” with moderate or large sample sizes (it is equal to logit for small sample sizes). For fixed effects models, probit and logit are equally good.
What is the disadvantage of logarithmic transformation?
Unfortunately, data arising from many studies do not approximate the log-normal distribution so applying this transformation does not reduce the skewness of the distribution. In fact, in some cases applying the transformation can make the distribution more skewed than the original data.