What is an example of central limit theorem?
Table of Contents
What is an example of central limit theorem?
Biologists use the central limit theorem whenever they use data from a sample of organisms to draw conclusions about the overall population of organisms. For example, a biologist may measure the height of 30 randomly selected plants and then use the sample mean height to estimate the population mean height.
What is the central limit theorem can you explain it with examples and why it is important?
The central limit theorem (CLT) states that the distribution of sample means approximates a normal distribution as the sample size gets larger, regardless of the population’s distribution. Sample sizes equal to or greater than 30 are often considered sufficient for the CLT to hold.
How do you explain the central limit theorem?
The central limit theorem states that if you have a population with mean μ and standard deviation σ and take sufficiently large random samples from the population with replacement , then the distribution of the sample means will be approximately normally distributed.

What are the three cases of the central limit theorem?
To wrap up, there are three different components of the central limit theorem: Successive sampling from a population. Increasing sample size. Population distribution.
What is the use of central limit theorem in solving problems involving sampling?
The Central Limit Theorem tells us that as sample sizes get larger, the sampling distribution of the mean will become normally distributed, even if the data within each sample are not normally distributed. We can see this in real data.
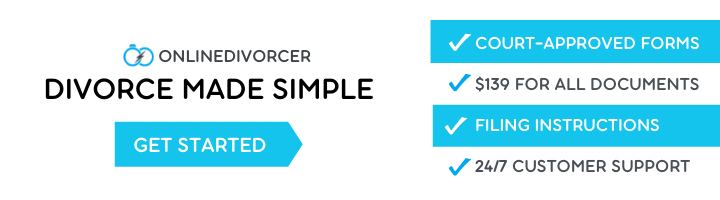
What is the main reason the central limit theorem is so useful?
Why is central limit theorem important? The central limit theorem tells us that no matter what the distribution of the population is, the shape of the sampling distribution will approach normality as the sample size (N) increases.
Why is CLT important?
The CLT performs a significant part in statistical inference. It depicts precisely how much an increase in sample size diminishes sampling error, which tells us about the precision or margin of error for estimates of statistics, for example, percentages, from samples.
Which of the following are correct general statements about the central limit theorem?
The correct general statements about the Central Limit Theorem is: 1. It specifies the specific shape of the curve which approximates certain sampling distributions.
When can we apply central limit theorem?
If the sample size is at least 30 or the population is normally distributed, then the central limit theorem applies. If the sample size is less than 30 and the population is not normally distributed, then the central limit theorem does not apply.
How many observations are needed for central limit theorem?
30 observations
In essence, this says that the mean of a sample should be treated like an observation drawn from a normal distribution. The Central Limit Theorem only holds if the sample size is “large enough” which has been shown to be only 30 observations or more.
What is the minimum sample size required for the central limit theorem?
30
Sample size equal to or greater than 30 are required for the central limit theorem to hold true. A sufficiently large sample can predict the parameters of a population such as the mean and standard deviation.
What is central limit theorem and its importance in statistics analysis?
What is the Central Limit Theorem? The CLT is a statistical theory that states that – if you take a sufficiently large sample size from a population with a finite level of variance, the mean of all samples from that population will be roughly equal to the population mean.
How is the central limit theorem used in statistical inference?
Central Limit Theorem (CLT) is an important result in statistics, most specifically, probability theory. This theorem enables you to measure how much the means of various samples vary without having to use other sample means as a comparison.
How do you know if central limit theorem apply?
Why is the CLT important?
What is the importance of the central limit theorem in real world studies?
Central limit theorem helps us to make inferences about the sample and population parameters and construct better machine learning models using them. Moreover, the theorem can tell us whether a sample possibly belongs to a population by looking at the sampling distribution.